Transdisciplinary Collaboration as a Vehicle for Collective Intelligence: A Case Study of Engineering Design Education*
ATILA ERTAS, JASMIN ROHMAN, PRATAP CHILLAKANTI and T. BATUHAN BATURALP
Texas Tech University, Department of Mechanical Engineering, Lubbock, Texas 79409, USA. E-mail: atila.ertas@ttu.edu
Abstract
The main objective of this paper is to introduce a new transdisciplinary collaborative research process model to develop collective intelligence through input from research teams as well as academic and non-academic experts. The paper discusses the need for new types of collaboration to solve complex problems and reviews the development of transdisciplinary approaches and collective intelligence. The paper proposes a transdisciplinary collaborative research process composed of three parts: (1) team building and collaborative problem understanding; (2) development of collective intelligence through interpretive structural modeling to classify the problem factors according to driving power and dependence, and (3) use of design structure matrices for transdisciplinary assessment and knowledge integration to form project teams. The proposed process model is illustrated through an example of a rural eco-village system of systems (SoS) drawn from the context of a senior capstone design course.Keywords: transdisciplinary collaboration; information sharing; collective intelligence; interactive management; interpretive structural modeling; design structure matrix; eco-village
1. Introduction
Over recent years, there has been increasing interest among researchers and educators for organized collaboration on the national and international levels. The evolution of large scale collaborative efforts between researchers from diverse disciplines was spawned from the necessity to increase efficiency in solving complex problems that require knowledge from multiple disciplines. Realizing the benefits from collaborative research, numerous initiatives have been launched to develop transdisciplinary research groups and establish new or larger centers of excellence [1–3].
One of the most constructive forms of knowledge transfer, for all partners involved, is Joint development of technologies, where companies and universities work together in pursuit of research objectives that are of mutual benefit, and are jointly managed under a collaborative agreement. In comparison with consultancy, contracts, or grants collaborative research is more interactive in nature and has the potential to beneficially persist for longer durations.
Disciplines developed over the last few centuries are convenient for organizing information and for basic educational activities; however, approaching complex problems and developing complex social–technical systems to address such problems requires a transdisciplinary approach. Complex problems by definition do not observe disciplinary boundaries, and effective collaboration on any level requires facilitation via an organizational structure.
Because of the following reasons collaboration on research and educational activities are not only useful, but imperative [4]:
Collaboration accelerates the pace of new discoveries and the expansion of human knowledge.
Innovation of technologies occurs through global cross communication.
Transdisciplinary collaboration in large-scale multi-national partnerships initiates speedy exploitation of promising results.
Research partnerships are essential in solving complex problems and to optimize knowledge acquisition.
Collaborative, transdisciplinary educational activities can better prepare today’s students to solve the complex problems that face mankind.
Collaboration among people from different nations in research and education can substantially benefit all parties involved. In order to meet new challenges in the 21st century, universities around the world are seeking to broaden their engineering education systems. In the near future, engineering education will be an international enterprise.
Hence, institutions of different nations must work together.
The world is constantly growing smaller and smaller. Challenging technical, medical, social, and cultural issues are no longer just local concerns—issues and problems must more and more be considered from a global perspective. Communication, collaboration, and education on a global scale are the keys to solving the complex problems and issues facing mankind in the 21st century. The successful development of a network of global collaboration would provide a common sharing of knowledge and benefit everyone by greatly increasing the ability to solve complex problems facing the peoples of the world. Such problems include living conditions, environmental issues, health and medical issues, energy issues, transportation issues, communication issues, and educational issues [4].
Although collaboration is not a new idea, new opportunities for collaboration exist in the 21st century’s global body of knowledge and information-driven economy. Technology has reduced distances in time and space while enhancing communication, hence opening the possibilities of exploring new boundaries in the area of international Transdisciplinary (TD) collaboration. Such international collaboration requires not only active participation of faculty but also participation of the top administrators of educational institutions.
Faculty and administrators should find innovative ways to work together across international boundaries. Recent advances in information and communications technologies are providing new opportunities for international cooperation in education and research [4, 5].
2. Transdiscipline
An interdisciplinary methodology has been defined as ‘‘two or more disciplines which combine their expertise to jointly address an area of common concern’’ (Davies & Devlin [6]). Ertas, et al., [7] indicated that interdisciplinarity forces one to ‘‘think across, beyond, and through the academic disciplines to encompass all types of knowledge about an idea, issue, or subject.’’
The creative potential of cross-disciplinary research increases as one move from interdisciplinary to transdisciplinary approaches [8]. The criteria of novelty and importance appear to differentiate transdisciplinarity from interdisciplinarity, which is the integration of insights from multiple disciplines. Intelligence and creativity provides a connection to transdisciplinarity [9].
Many scientists and engineers made excellent contributions to the development of a transdisciplinary methodology since 1970s [10–14]. Klein states that the term transdisciplinarity signifies a new structure of unity informed by the world view of complexity in science, a new mode of knowledge production that draws on expertise from a wider range of organizations, and collaborative partnerships [15]. Hadorn, H. G. et al., underline that transdisciplinary research transgresses boundaries between scientific disciplines and between science and other societal fields and includes deliberation about facts, practices and values [16]. There is a need for transdisciplinary research when knowledge about a societally related problems are uncertain, when the concrete nature of problems is disputed, and when there is a great deal at stake for those concerned by problems and involved in dealing with them [17].
‘‘Transdisciplinarity is a practice of acquiring new knowledge through education, research, design, and production with a broad emphasis on complex problem solving. The goal of transdisciplinary practice is to improve our understanding of complex issues by extracting the valuable aspects of typical academic disciplines and thereby generate both a more integrative and universal solution to support an issue of importance to society’’ [18].
3. Transdisciplinary collective intelligence
Transdisciplinary collaboration is essential to solve society’s ‘‘messy’’ or ‘‘wicked’’ problems, e.g., those with inter-connected influences and requirements such that the proposed solution causes other problems, i.e., climate change; response to natural disasters; enhanced national security [19]. Transdisciplinary collaboration has a big potential to speed the rate at which research can contribute to the understanding of the problem, accelerate the pace of new discoveries, and expand human knowledge.
Transdisciplinarity provides a good framework and add to the current approaches for collective intelligence. Transdisciplinary collective intelligence is a new mode of information gathering, knowledge creation, and decision-making that draws on expertise from a wider range of organizations (academic or non-academic) and collaborative partnerships.
Fig. 1 shows the proposed TD research process model which is conceptualized in three phases: 1) collaboratively defining the research problem objective(s) and building a collaborative research team; 2) developing collective intelligence to solve complex problems in question; and 3) TD assessment and knowledge integration.
3.1 Team building and collective understanding of the research problem
TD-teams can be developed with distributed leadership—team leadership can change in accordance with the particular expertise required. Most of the time, structuring and understanding of a complex problem may become problematic—collaborating team members may not even agree on what the problem is and no solution can make everyone happy [20]. Considering a hypothetical example, imagine students in a senior design class working on a research project for a class. A sample research project might be to design and develop ideal selfsustaining rural eco-village systems of system (SoS) (approximately 100 people live in independent 25 homes).
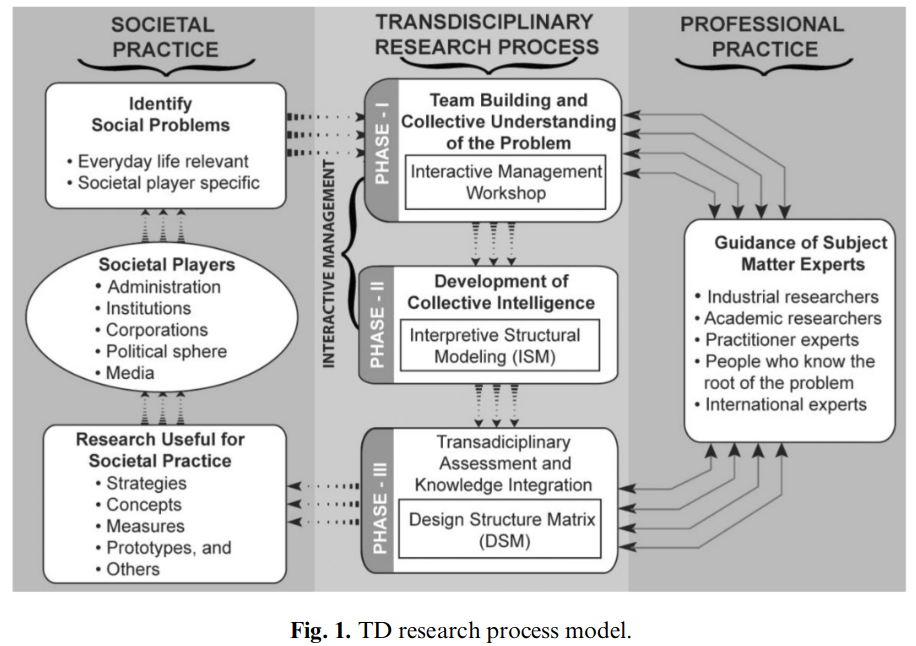
3.1.1 Interactive management (IM) workshop
Assume that research sub-project teams of four with specific roles for each sub-project teams are considered. Depending on the assigned task, each subproject team may have minimum of three and maximum of five students. Using any communication platform, a workshop can be organized where sub-project teams will introduce their project proposals (concepts) about the project being explored.
Through dialog, the collective best ideas of subproject teams will emerge and the incorrect or fuzzy ideas that teams held at the outset will be recognized as incorrect or sharpened to make them useful. Through the Interactive Management (IM) process [21], ideas with high interaction will be grouped into clusters. Thus, team members can identify and examine cluster interactions internally and interactions between clusters. Clusters will be placed in a sequence by using Interpretive Structural Modeling (ISM) [19]. It is important to note that the workshop coordinator should help the team members to understand the importance of team transactive memory process (team members in a group develop a shared system for encoding, storing, and retrieving information where each person is responsible for memorizing only part of the total information) [22].
Through Interactive Management (IM) Workshop sub-project team members will experience the mutual understanding and learning that are an integral part of the TD research process.
3.2 Development of collective intelligence-interpretive structural modeling
Interpretive Structural Modeling (ISM), a methodology for dealing with complex issues was proposed by Warfield in 1973. It is a computer-assisted learning process that provides fundamental understanding of how various parameters (elements, variables, system components, etc.) relevant to the problem or issue interrelated and thus helps researchers to structure them in a meaningful manner to develop collective intelligence to overcome challenging complex problems.
This interactive learning, information processing, and developing collective intelligence is especially useful for working in a group to develop a map of the complex relationships between various elements involved in a challenging complex issue. The relationship map includes paths of ideas and threads of thought to transform unclear and poorly expressed mental model of an issue into a visible, well defined, and relatively easily solvable model. The fundamental approach of this process is to use academic and non-academic experts’ practical experience and knowledge to decompose a complex issue into smaller sub-issues and build a simpler multilevel structural model. For the hypothetical example, general activities to build an Interpretive Structural Modeling are shown in Fig. 2. Note that the sequence of these activities may change from one application to another.
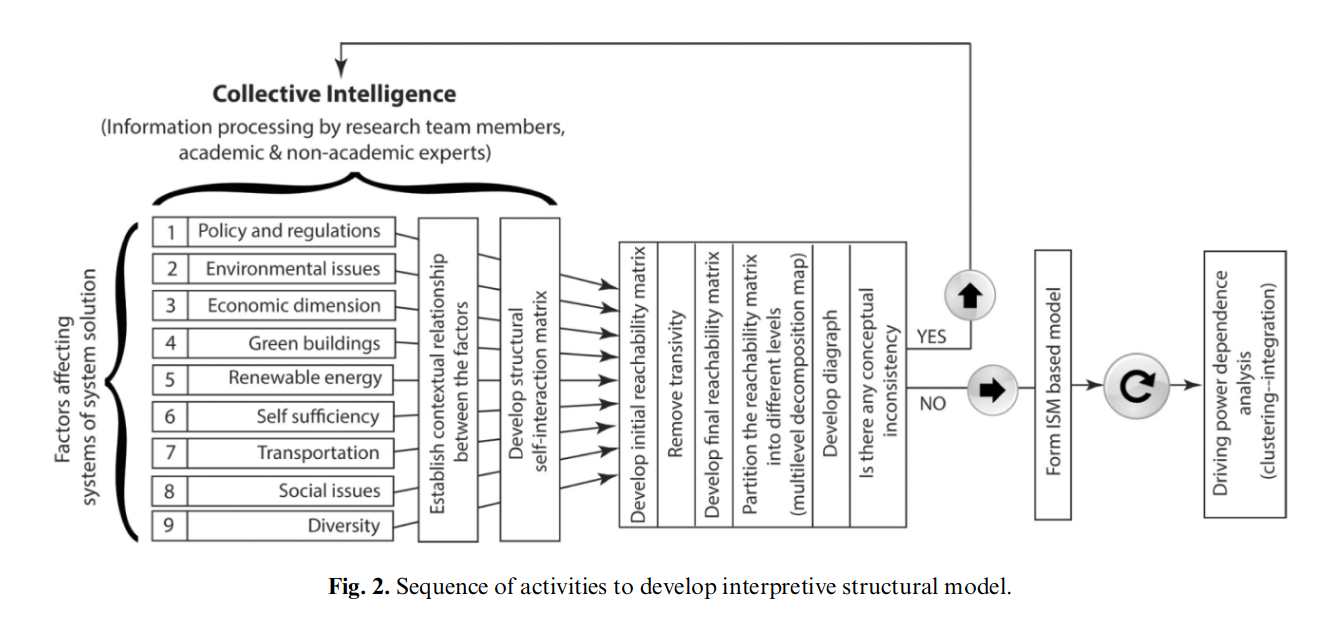
3.2.1 Sequence of activities to develop ISM model
The first step in ISM process is to organize a group of people with relevant knowledge, skills, and background. This group of team should consist of academic and non-academic experts from diverse disciplines (Fig. 1). A coordinator who will control the ISM process should not only be knowledgeable in the subject matter but also be familiar with the ISM process. At this stage, it is also important clearly identify the problem (issue) which will be studied as explained in section 3.1.1.
Second step is identify and define the factors effecting issue as shown in Fig. 2. Through brainstorming and consultation with the domain experts, research group members work together to document all the possible factors (elements) whose relationships are to be modeled. Then the most important factors will be identified for the model development.
Nominal Group Technique (NGT) is an efficient method for generating ideas for defining a set of factors [23]. Five basic steps of NGT process are given as: (a) clarification of a trigger question, (b) silent generation of ideas in writing by each group members, (c) round-robin recording of the ideas, (d) ongoing discussion of each idea for clarification and editing, and, (e) voting to obtain a preliminary ranking of the ideas in terms of significance.
Structural self-interaction matrix
The following step is to establish contextual relationship to develop structural self-interaction matrix (SSIM) shown in Fig. 3. Using expert opinions along with research team members SSIM is develop which shows the direction of contextual relationship among the factors effecting the issue or problem. Four symbols are used in this matrix are:
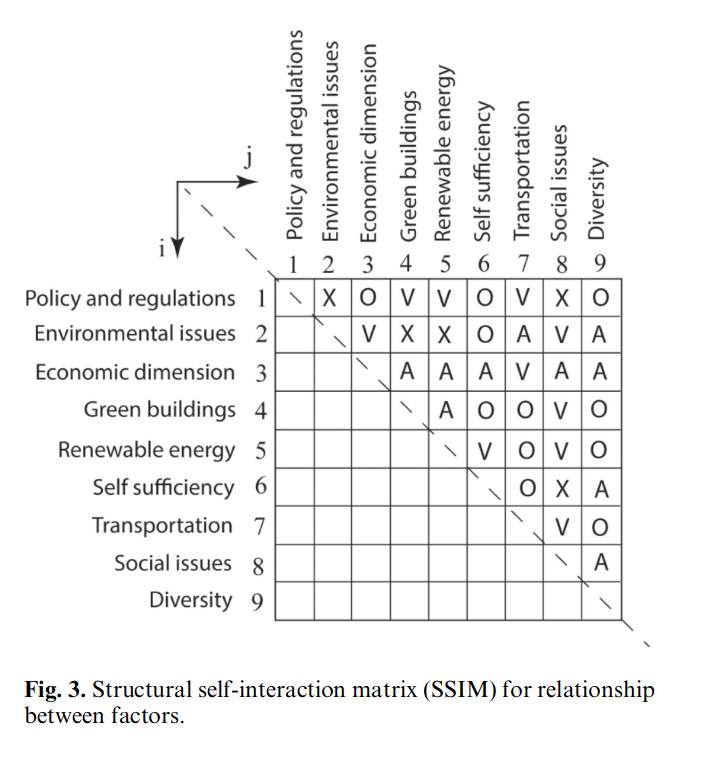
Enter V when the relation is from i to j (in the reachability matrix substitute in the (i,j) entry as 1 and (j,i) entry as 0).
Enter A when the relation is from j to i (in the reachability matrix substitute in the (i,j) entry as 0 and (j,i) entry as 1).
Enter X when the relation is from I to j and j to I, both direction (in the reachability matrix substitute in the (i,j) entry as 1 and (j,i) entry as 1).
Enter O when there is no relationship between the row (i) element and the column (j) element (in the reachability matrix substitute in the (i,j) entry as 0 and (j,i) entry as 0).
In developing structural self-Interaction matrix, if the relationship between factors is week, it is assumed that there is no relationship between factors. Enter A when the relation is from j to i (in the reachability matrix substitute in the (i,j) entry as 0 and (j,i) entry as 1).
Enter X when the relation is from I to j and j to I, both direction (in the reachability matrix substitute in the (i,j) entry as 1 and (j,i) entry as 1).
Enter O when there is no relationship between the row (i) element and the column (j) element (in the reachability matrix substitute in the (i,j) entry as 0 and (j,i) entry as 0).
Adjacency matrix
Then the adjacency matrix, Ra shown in Fig. 4 is developed by transforming SSIM into a binary matrix by substituting V, A, X, and O by 1 and 0 per the schema described above in the matrix that reflects the directed relationships between the elements. This matrix answers the question of ‘‘yes’’ or ‘‘no’’. In other words, starting from factor (i), can factor (i) reach to factor (j) to contribute and improve? If so, substitute in the (j) entry as 1 and (i) entry as 0). This means that there is a walk from (i) to (j). Main diagonal of reachability matrix is filled with ones.
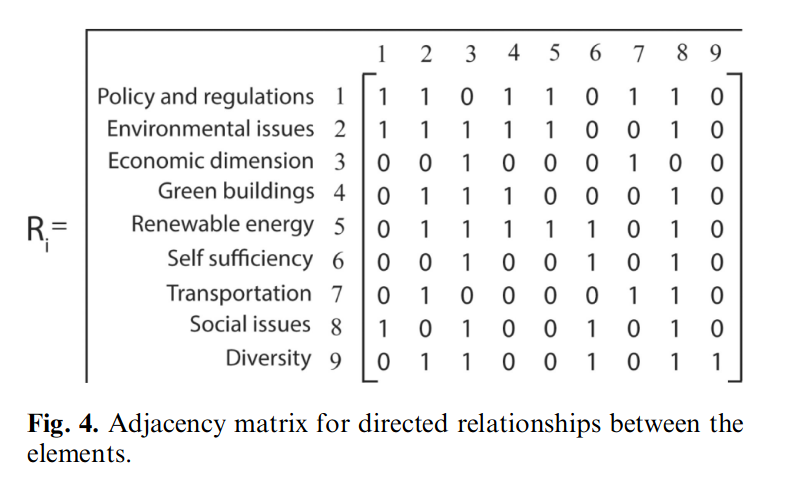
Reachability matrix with transivity
The reachability matrix with transivity Rt is shown in Fig. 5. This matrix is checked for the transivity rule and will be updated until transivity is established. Transivity rule states that, if a factor (element) A is related to factor B and if factor B is related to factor C, then factor A is related to factor.
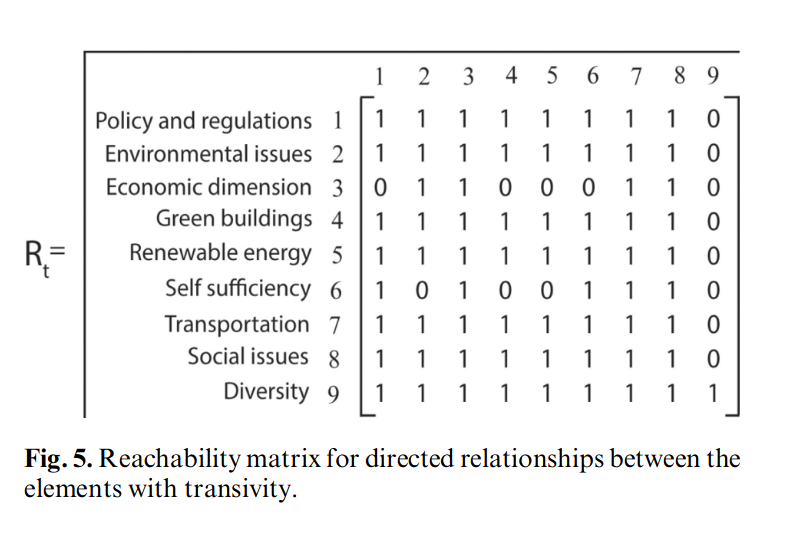
C. Following transivity rule a reachability matrix which considers transivity is developed.
Final reachability matrix
Driving power and dependence of factors are also computed in the final reachability matrix as shown in Fig. 6. Summation of ones in the corresponding rows gives the driving power and the summation of ones in the corresponding columns gives the dependence.
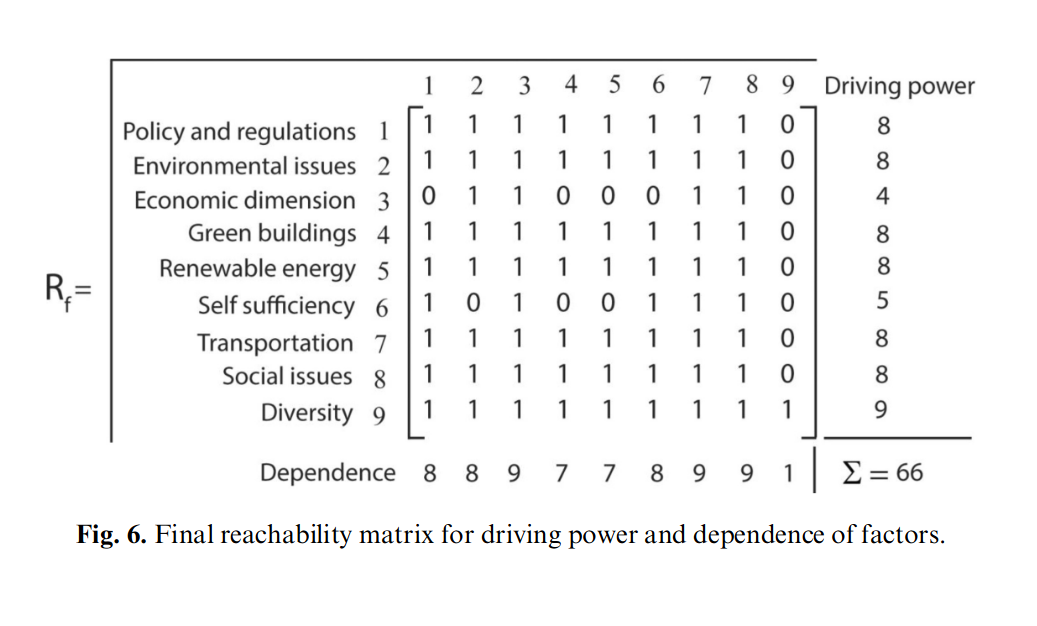
Level partition
For level partition, the final reachability matrix, Rf along with antecedents of each element of prospects are used [24]. Previously obtained driving force and dependence helps to classify the factors into groups. These groups’ positions are determined by the separation of antecedent set and reachability set. From these two sets intersection set is determined. The factors are common in the reachability set and antecedent set are included in the intersection set. These three sets help to identify the level of the factors.
If all the factors of the intersection and reachability sets of any particular factor are the same, then that factor is identified as the top level group (level I group)
in the ISM hierarchy. Once the top level factors are identified, it is removed from the set to identify the next level. This iteration process is repeated until all the levels are identified. As shown in Table 1, factors 3, 8, and 9 are found to be at level I. Hence, it will be positioned at the top level group in the ISM hierarchy. This process is repeated until all the levels are determined. The factors along with their reachability set, antecedent set, intersection set and levels, are shown in Tables 1, 2, 3, and 4. These levels help to build the diagraph and ISM model. 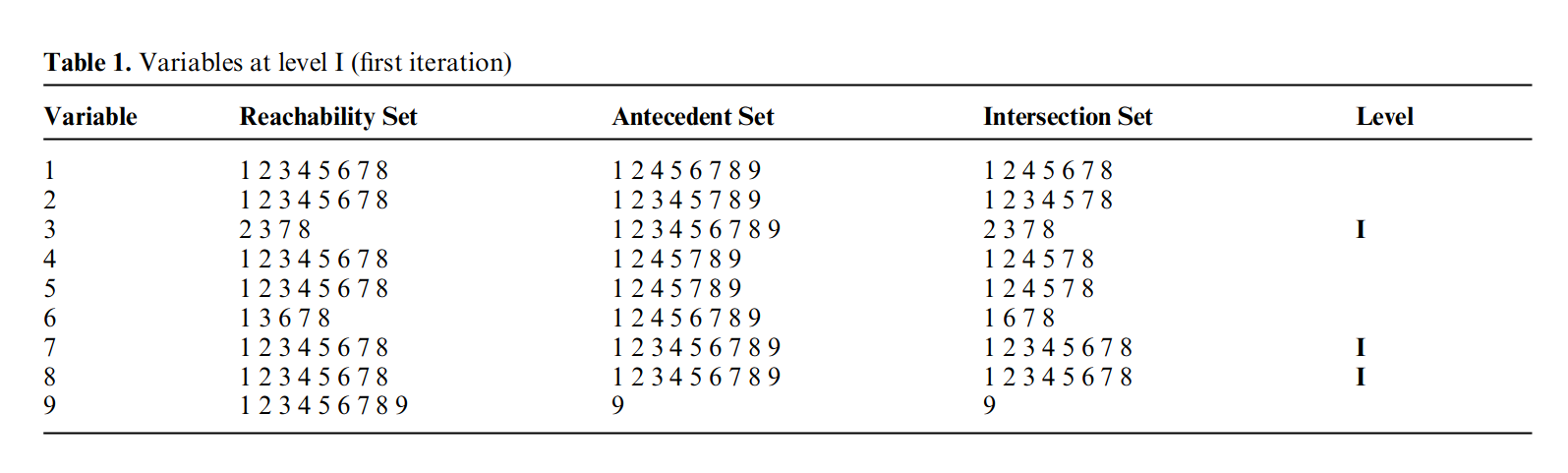
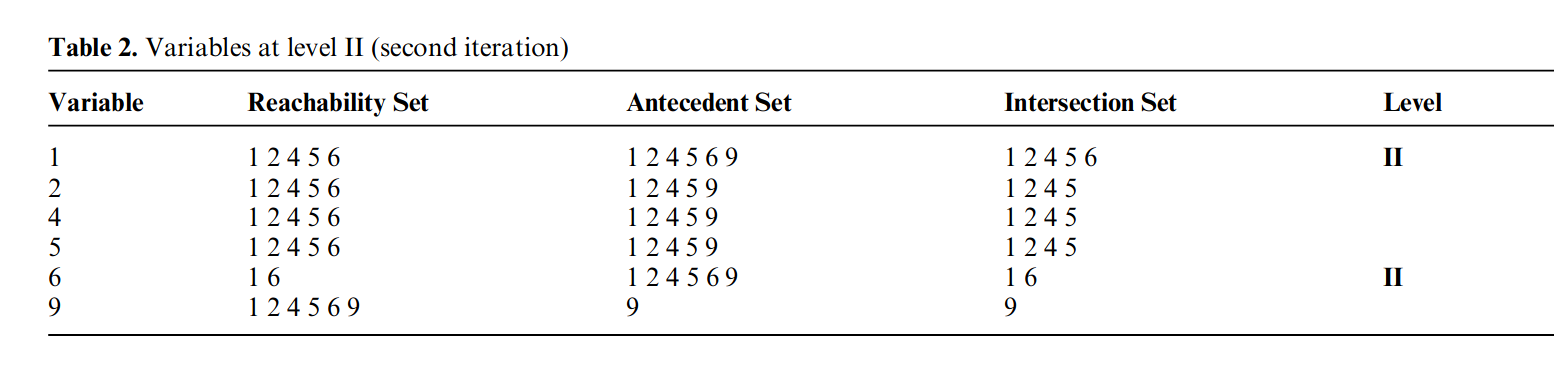
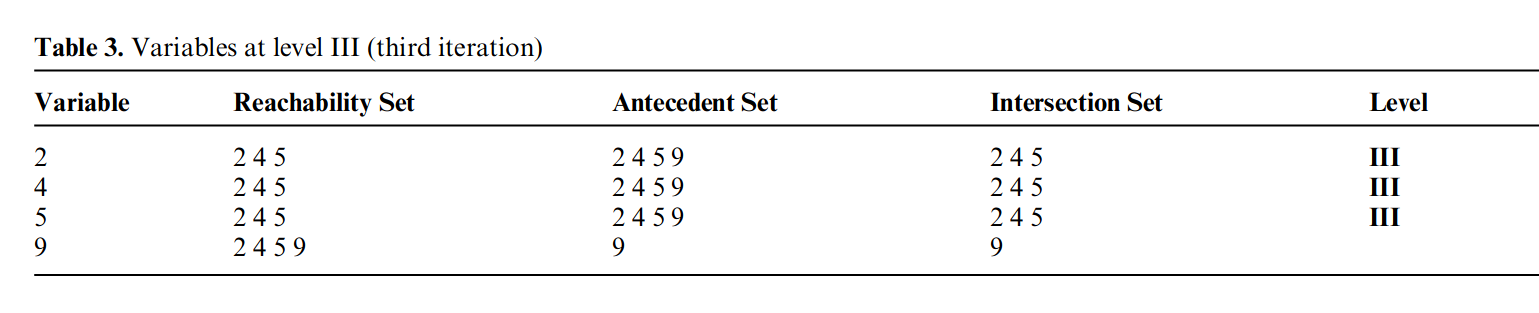

Formation of digraph and ISM based models As shown in Fig. 7, the association of sets and binary relations through matrices can now be converted into graphical form by using theory of digraphs (directed graphs) [25]. If there is a relationship between factors j and i, connection between factors will go from i to j. Finally, digraph is converted to ISM to see a broad representation of the interrelationship between the factors (Fig. 8).
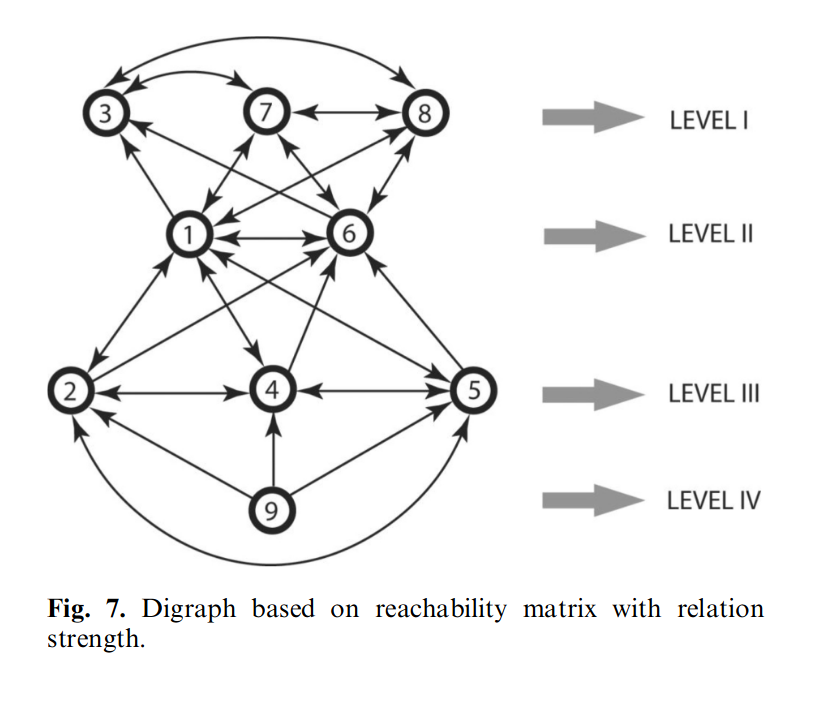
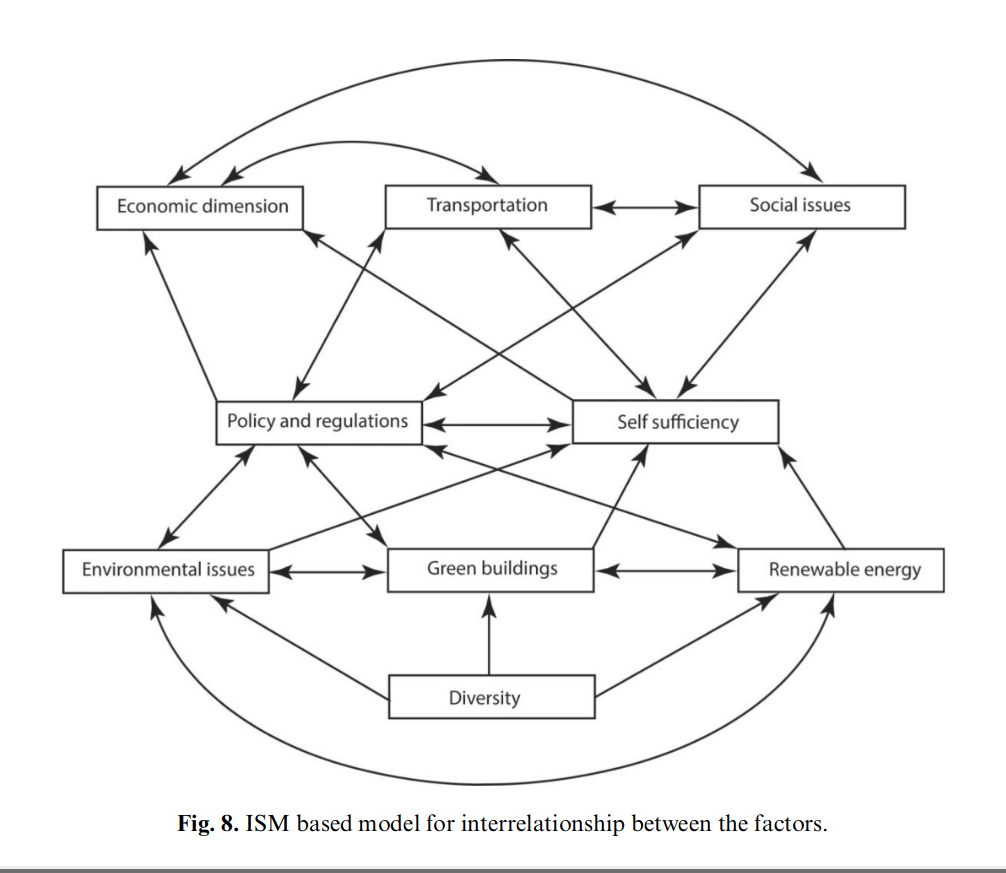
MICMAC analysis—classification of performance measures
Performance goals for the eco-village may include: reduction of energy (using green buildings, wind and solar energy), water, land, and natural resource management, reduction of water use, waste treatment, recycling, roof-top capture of rainwater for landscape irrigation and production of fruits and vegetables guided by intertwined educational, economical, environmental, sustainable and social goals. MICMAC was developed by Duperrin and Godet in 1973 to study the diffusion of impact through reaction paths and loops for developing hierarchies for members of an element set [26]. The purpose of MICMAC analysis is to arrange the factors with respect to their driving power and dependence in four clusters [27]: (1) autonomous, (2) dependent, (3) linkage, and (4) independent factors. The driving power and dependence of each of factors are imported from Fig. 6. Fig. 9 shows the driving power-dependence diagram for self-sustaining rural eco-village SoS.
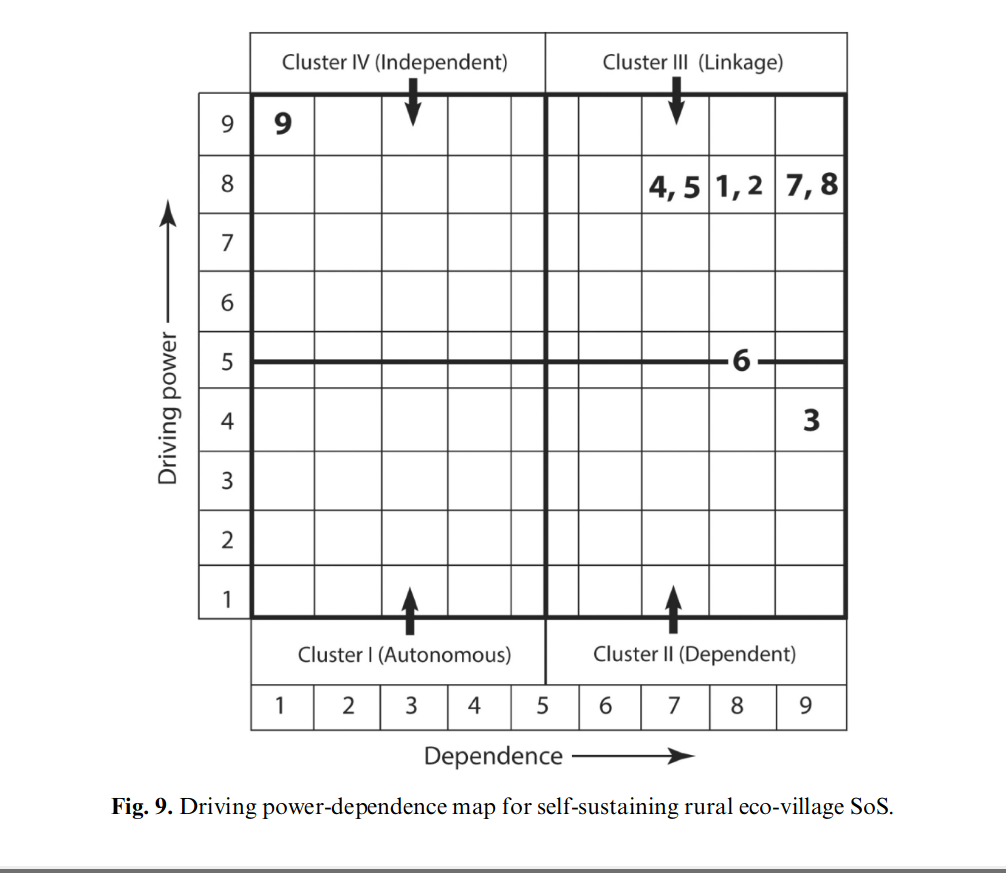
MICMAC分析Matrix Impact Cross-Reference Multiplication Applied to Classification 是一種用於評估和分類組織內部各種因素相互影響和依賴關係的方法。這種分析通常用於確定哪些績效指標或實踐具有推動力(即對其他因素有強烈影響)以及哪些是依賴性的(即受其他因素影響較大)。
舉例來說,假設一家製造公司想要提升其運營績效,它可能會識別出影響運營績效的關鍵實踐,如精益管理、組織文化、ISO 9001質量管理體系和人力資源管理實踐。這些實踐被視為具有強大推動力的因素,因為它們對公司的運營績效有顯著影響。
在MICMAC分析中,這些實踐會被分類為不同的群組,以展示它們之間的相互影響和依賴關係。例如,精益管理可能會直接影響生產效率和質量控制,而組織文化可能會影響員工的工作滿意度和創新能力。通過這種分析,公司可以更好地理解各種實踐之間的關係,並確定哪些實踐是改善運營績效的關鍵驅動因素。
這種分析的結果可以幫助公司決定在戰略和運營層面上應該重點關注哪些實踐,以及如何有效地整合這些驅動實踐,從而在有限的資源下提高運營績效。這種策略性的理解對於研究人員和實踐者來說都是非常有價值的,因為它可以幫助他們識別重點領域,並更有效地應對這些驅動實踐,從而在很大程度上影響運營績效。
參考資料:Boosting Up Operational Performance of Manufacturing Organizations through Interpretive Structural Modelling of Enabling Practices
MICMAC分析將因素分為四個群組,每個群組代表因素的不同特性和相互關係:
1. Cluster I (Autonomous): 自主群組的因素通常對其他因素的影響較小,同時也不太受其他因素的影響。這些因素在系統中相對獨立,並且在整體結構中不是非常關鍵。
2. Cluster II (Dependent): 依賴群組的因素受到其他因素的強烈影響,但它們對其他因素的影響力較弱。這些因素通常是結果或輸出,它們的表現依賴於其他因素。
3. Cluster III (Linkage): 連結群組的因素既受到其他因素的影響,也對其他因素有顯著的影響力。這些因素在系統中扮演重要的中介角色,因為它們連接了系統的不同部分。
4. Cluster IV (Independent): 獨立群組的因素對其他因素有強烈的影響力,但它們自身不太受到其他因素的影響。這些因素通常是驅動因素,它們推動系統的變化和發展。
製作MICMAC分析表的內容規則包括:
• 識別因素: 首先,需要識別出所有相關的因素,這些因素可能會影響系統的績效或結果。
• 評估影響力和依賴性: 然後,評估每個因素對其他因素的影響力(推動力)以及它們對其他因素的依賴性。
• 建立交叉影響矩陣: 使用交叉影響矩陣(Cross-Impact Matrix)來分析因素之間的相互影響。
• 進行分類: 根據因素的推動力和依賴性,將它們分類到上述四個群組中。
• 分析和解釋: 最後,分析這些群組之間的關係,並解釋每個群組對系統的意義和影響。
這種分類方法有助於組織或研究者理解不同因素之間的動態關係,並確定哪些因素是關鍵驅動因素,哪些是潛在的風險點,以及哪些因素需要進一步的關注和管理。這有助於優化資源分配,並制定更有效的策略和決策。這種分析通常與解釋結構模型(Interpretive Structural Modeling, ISM)一起使用,以視覺化地呈現因素之間的層次和關係。這些工具和方法在許多領域都有應用,如組織管理、風險評估、政策制定等。
舉例來說,假設一家製造公司想要提升其運營績效,它可能會識別出影響運營績效的關鍵實踐,如精益管理、組織文化、ISO 9001質量管理體系和人力資源管理實踐。這些實踐被視為具有強大推動力的因素,因為它們對公司的運營績效有顯著影響。
在MICMAC分析中,這些實踐會被分類為不同的群組,以展示它們之間的相互影響和依賴關係。例如,精益管理可能會直接影響生產效率和質量控制,而組織文化可能會影響員工的工作滿意度和創新能力。通過這種分析,公司可以更好地理解各種實踐之間的關係,並確定哪些實踐是改善運營績效的關鍵驅動因素。
這種分析的結果可以幫助公司決定在戰略和運營層面上應該重點關注哪些實踐,以及如何有效地整合這些驅動實踐,從而在有限的資源下提高運營績效。這種策略性的理解對於研究人員和實踐者來說都是非常有價值的,因為它可以幫助他們識別重點領域,並更有效地應對這些驅動實踐,從而在很大程度上影響運營績效。
參考資料:Boosting Up Operational Performance of Manufacturing Organizations through Interpretive Structural Modelling of Enabling Practices
MICMAC分析將因素分為四個群組,每個群組代表因素的不同特性和相互關係:
1. Cluster I (Autonomous): 自主群組的因素通常對其他因素的影響較小,同時也不太受其他因素的影響。這些因素在系統中相對獨立,並且在整體結構中不是非常關鍵。
2. Cluster II (Dependent): 依賴群組的因素受到其他因素的強烈影響,但它們對其他因素的影響力較弱。這些因素通常是結果或輸出,它們的表現依賴於其他因素。
3. Cluster III (Linkage): 連結群組的因素既受到其他因素的影響,也對其他因素有顯著的影響力。這些因素在系統中扮演重要的中介角色,因為它們連接了系統的不同部分。
4. Cluster IV (Independent): 獨立群組的因素對其他因素有強烈的影響力,但它們自身不太受到其他因素的影響。這些因素通常是驅動因素,它們推動系統的變化和發展。
製作MICMAC分析表的內容規則包括:
• 識別因素: 首先,需要識別出所有相關的因素,這些因素可能會影響系統的績效或結果。
• 評估影響力和依賴性: 然後,評估每個因素對其他因素的影響力(推動力)以及它們對其他因素的依賴性。
• 建立交叉影響矩陣: 使用交叉影響矩陣(Cross-Impact Matrix)來分析因素之間的相互影響。
• 進行分類: 根據因素的推動力和依賴性,將它們分類到上述四個群組中。
• 分析和解釋: 最後,分析這些群組之間的關係,並解釋每個群組對系統的意義和影響。
這種分類方法有助於組織或研究者理解不同因素之間的動態關係,並確定哪些因素是關鍵驅動因素,哪些是潛在的風險點,以及哪些因素需要進一步的關注和管理。這有助於優化資源分配,並制定更有效的策略和決策。這種分析通常與解釋結構模型(Interpretive Structural Modeling, ISM)一起使用,以視覺化地呈現因素之間的層次和關係。這些工具和方法在許多領域都有應用,如組織管理、風險評估、政策制定等。
3.3 TD assessment and knowledge integration
Transdisciplinary assessment (TA) includes integrating people (social), artefacts (technical), and knowledge (cognitive) associated with different scientific and non-scientific knowledge domains [28] into an appropriate methodology [29]. Societal players who are affected by a problem along with the research team must be drawn into the research process to effect scientifically valid research (Fig. 1).
In the third phase of TD research process, design structure matrix (DSM) is used for SoS design and development. DSM provides a simple, visible, well defined representation of complex systems that aids meaningful solutions to decomposition and integration problems [30, 31]. In order to reduce the technical complexity of eco village SoS research project, work will be distributed among several design and development student teams. The following steps are used to reorganize student research teams:
1.Decompose the research team into sub-project teams with specific functions, roles, or assignments—map the sub-project teams to product components.
2.Document the interactions between the teams.
3.Analyze potential reintegration of the teams via clustering (integration analysis)
Decomposition of research team into sub-project teams creates a crucial managerial challenge due to the complex web of interactions between teams and team members for design and development of eco village SoS. This effort can be coordinated and orchestrated by the system team. The system team manages the whole design and development process, interacts with all the sub-project teams, and provides guidance and ensures that the systems’ compatibility with each other within the system of systems exists [32].
Complex systems such as SoS in this case, require communications and exchange of information among team members and teams. Information flow between team members can be created using a team-based DSM in order to better map teams, to address the SoS problems. Clustering (integration) of teams can provide new understandings into system decomposition and integration. To optimize the team collaboration for collective intelligent (knowledge integration through transactive memory process), interactions between teams within DSM clusters should be maximized and interactions between DSM clusters should be minimized [33]. While minimizing the size of the clusters some overlapping between the clusters is also recommended [34].
4. Discussions
The hypothetical example of an ideal self-sustaining rural eco-village systems of system (SoS) in a multiproject environment given in this paper can be created by optimizing natural resources—using renewable energy, growing much food as possible through intensive agriculture, community building, sharing common resources, and finally ‘‘all for one and one for all’’.
Factors in designing and developing an ideal selfsustaining rural eco-village SoS shown in Fig. 2 is simplified by lumping similar factors serving to same purposes. Such as renewable energy may include solar, wind, hydro energies. Environmental issues may include recycling, air, water, and soil protection. Self-sufficiency may include recycling, and sharing common resources (food production etc.), Diversity may include age, income levels, culture, color, being from different country, and social issues may include encouraging unity through diversity, fostering cultural expression, emphasizing holistic health practices, being committed to living in a community. Designing and developing an ideal SoS is not an easy task. Many subsystems (e.g., energy, green building, transportation, recycling, etc.) must be integrated to achieve an overall optimum SoS solution.
The main objective of this paper is to introduce a new TD research proses model in education which deals with such complex system development by creating and using collective intelligence through transdisciplinary collaborative effort. Interpretive Structural Modeling (ISM), a methodology for dealing with complex SoS design and development is the key component of this research. Building collective intelligence to understand how factors affecting SoS performance and their relationships are an important part of interpretive structural modeling.
After removing the transivities based on the reachability matrix as described in the ISM approach, the digraph (Fig. 7) is converted into the ISM and finally the structural hierarchy of the performance measure factors of SoS is developed as shown in Fig. 8. This figure depicts visually the direct and indirect relationships between the factors affecting the performance of ideal self-sustaining rural eco-village systems of system.
Two main important factors, self-sufficiency (measure 6) and policy and regulations (measure 1) shown in Figs. 7 and 8 are the most preliminary factors which need to be analyzed before other factors as most of the other factors depend on them. These two factors not only affect but also they are affected by the other factors. For selfsufficient eco village, the main goal must be a very high level of self-sufficiency and a very small ecological footprint. However, in some areas, laws and regulations can be major barriers for rural eco village development.
As shown in Figs. 7 and 8, factors economic dimension (measure 3), transportation (measure 7), and social issues (measure 8) are positioned at the top of the hierarchy. They are also very significant measures for the development of a rural eco village. Of course, one of the main reasons behind creating eco villages is families (social), rural eco village must have connection to the other world (transportation), and a true economy that is resulting from the value of people and benefited by balanced use of land (economic dimension). These three factors are strongly interrelated—affecting and affected from each other. These higher level factors have greater influence on the eco village SoS.
Hence, when designing and developing such SoS, these three factors can be first evaluated and kept constant as much as possible during the performance improvement. In other words, first make sure that transportation issues are solved, within certain limitations economic modeling is developed, and social issues are resolved, and then start iteration by changing the other remaining factors to find the best possible solution for the ideal self-sufficient eco village systems of system.
As shown in Fig. 9, all performance measures of factors effecting SoS have been classified into four categories. Cluster I includes autonomous factors.
As seen in Fig. 9, they have low driving power and low dependence, hence they can be eliminated from the SoS. For this case, no factor has been identified as an autonomous factor. This indicates that there is no disconnected factor from the SoS.
Cluster II includes dependent factors that have low driving power and high dependence. As seen in Fig. 9, only economic dimension (measure 3) has a smaller guidance power but it is extremely dependent to the SoS— this factor may not affect other factors but it is affected by other factors of SoS.
The factors in the linkage cluster have to be given extreme importance due to their high driving power and high dependence power. Cluster III includes linkage factors of policy and regulations (measure 1), environmental issues (measure 2), green buildings (measure 4), renewable energy (measure 5), selfsufficiency (measure 6), transportation (measure 7), and social issues (measure 8). As being the most important factors, they affect and depend on other factors in SoS. Any action on any of the factor in cluster III will affect the entire SoS. Therefore, as mentioned earlier, designing such systems of system is a challenging task and seven major factors within this cluster which influence the SoS performance must be carefully analyzed and integrated with the whole projected system. Note that, self-sufficiency is on the border of the dependent and linkage cluster. This factor, not only can be affected by other factors of SoS but also it can affect the other factors. Cluster IV includes independent factor of diversity (measure 9) with a strong drive power but very week dependence. This factor is the key driver for the eco-village SoS performance. Diversity is the strength and an important element. As diversity has impact on many factors, eco-village participants have to pay maximum attention to establish a diverse but integrated community for peaceful, healthy, and sustainable life so that issues will not be out of control.
5. Conclusions
In this paper, the author introduces a new transdisciplinary collaborative research process model in education for the purpose of developing collective intelligence through input from research teams, academic, and non-academic experts. The paper motivates the need for new types of collaboration to solve complex problems and discusses the development of transdisciplinary approaches and models of collective intelligence. The author proposes a transdisciplinary collaborative research process composed of three parts. First, team building and collaboration are used for problem understanding;
Second, Collective intelligence is developed through structural modeling to classify problem factors according to driving power and dependence. Eco-community determines the course of human interaction, the prospects for the impact on the natural environment, social issues, and sustainable peaceful, healthy life. Diverse participants in an ecovillage may have their personal and collective choices and may have an enormous impact on their interaction in cooperative and competitive modes.
Developing self-sufficient eco village SoS in this paper is a hypothetical case study. Moreover, information processing to develop structural self-interaction matrix shown in Fig. 3 was based on few experts’ opinions. The results of model analysis may differ in real world setting. Of course, capability of a group of team members along with academic and non-academic experts collectively can envision a better and different solution model for challenging complex problem such as this one.
It is important to note that ISM is a tool to identify the order and directions of the complexity of relationships among the system factors (elements). It does not provide any relative weight associated with the factors.